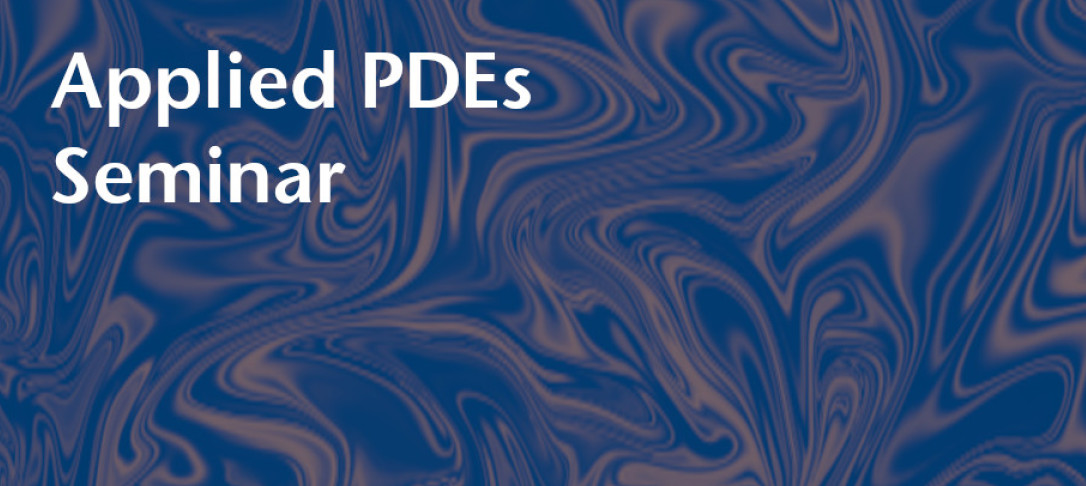
In this talk, we discuss some interesting problems and recent results on the interface of deep learning, approximation theory and control theory. Through a dynamical system viewpoint of deep residual architectures, the study of model complexity in deep learning can be formulated as approximation or interpolation problems that can be studied using control theory, but with a mean-field twist. In a similar vein, training deep architectures can be formulated as optimal control problems in the mean-field sense. We provide some basic mathematical results on these new control problems that so arise, and discuss some applications in improving efficiency, robustness and adaptability of deep learning models.