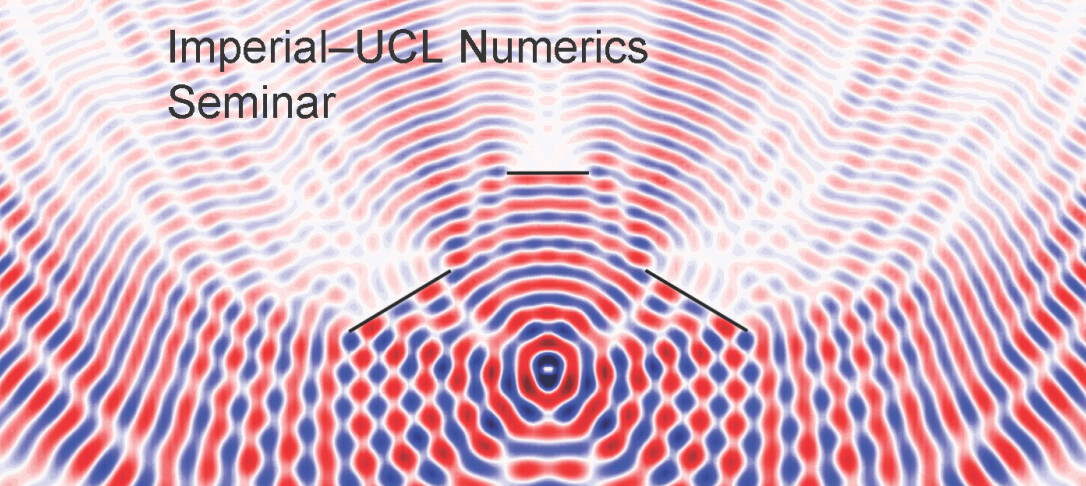
Multi-level Nonlinear Optimal Control with Neural Surrogate Models
Optimal actuator and control design is studied as a multi-level optimization problem, where the actuator design is evaluated based on the performance of the associated optimal control. The evaluation of the optimal control for a given actuator realisation is a computationally demanding task, for which the use of a neural network surrogate is proposed. The use of neural network surrogates to replace the lower level of the optimization hierarchy enables the use of fast gradient-based and gradient-free consensus-based optimization methods to determine the optimal actuator design. Through further study of the optimality conditions, we enhance the surrogate model leveraging Sobolev training, allowing for scenarios with limited training data. The effectiveness of the proposed surrogate models and optimization methods is assessed in tests related to optimal actuator location for heat and Burger’s equation control.