Friday 21 May Schedule
Information-Theoretic Methods in Distributed Learning
- 13:00: Keynote talk by Mohammad Ali Maddah-Ali (Nokia Bell Labs)
- Title: Coalition Through Coding: New Opportunities and Threats
Abstract
Information systems, running distributed algorithms and platforms, such as distributed learning, distributed storage, and distributed ledgers (blockchain), raise a wide range of challenges in terms of the security of the network, the privacy of the data, and the accuracy of the results. Working with coded data is known as an effective approach to alleviate those challenges. In this talk, we will review some of the coded solutions, and argue that while coding can help, it can create some serious, but overlooked, inefficiencies and security threats. This is particularly the case where the computation is complicated (e.g., training deep neural networks), the system is multi-user (e.g., multi-user secret sharing), or the encoding has to be distributed (e.g., sharded blockchains). We will also show how to bound and mitigate the new threats and inefficiencies, and discuss open problems.
- 14:00: Invited talk by Lifeng Lai (University of California Davis)
- Title: Distributed Statistical Inference with Compressed Data
Abstract
In the classic statistical inference problems, all data is available at a centralized location. With the explosion of the size of data sets, it is increasingly common that data is stored in multiple terminals connected by links with a limited communication capacity. In this scenario, terminals have to exchange compressed data and perform statistical inference using the compressed data. In this talk, we will discuss our recent work that addressed the following two questions: 1) Suppose we would like to achieve the same optimal inference as that of the centralized case, how much data compression can be performed?; and 2) Suppose we compress the data extremely (zero-rate compression), what is the optimal inference performance?
- 14:20: Invited talk by Songze Li (HKUST)
- Title: DeepPrivate: Scalable Distributed DNN Training with Data and Model Privacy
Abstract
A key friction in the information era is between the high utility of data and stringent privacy considerations. One possible resolution is to perform distributed learning with privacy guarantee. Consider N nodes, each of which stores locally some (function of) data from a set of clients, collaboratively train a model that is requested by a remote learner node, using data from all clients collectively. For this task, we develop DeepPrivate, a protocol that provides information-theoretic guarantees simultaneously for data and model privacy against any T < N colluding workers and data privacy against the curious learner. To achieve this, DeepPrivate partitions the entire dataset into K < N parts, and leverages secret sharing and compatible erasure coding to securely distribute the coded data partitions and intermediate computation results during the training process. As a result, DeepPrivate achieves a factor $K = \Theta(N)$ reduction in computation complexity compared with state-of-the-art private machine-learning frameworks. Furthermore, DeepPrivate improves the scalability and security via layer-by-layer training, which allows the workers to only compute polynomials of low degree during training, at a small cost on the model accuracy.
- 14:40: Invited talk by Gauri Joshi (Carnegie Mellon University)
- Title: Adaptive Quantization of Model Updates for Communication-Efficient Federated Learning
Abstract
Communication of model updates between client nodes and the central aggregating server is a major bottleneck in federated learning, especially in bandwidth-limited settings and high-dimensional models. Gradient quantization is an effective way of reducing the number of bits required to communicate each model update, albeit at the cost of having a higher error floor due to the higher variance of the stochastic gradients. In this work, we propose an adaptive quantization strategy called AdaQuantFL that aims to achieve communication efficiency as well as a low error floor by changing the number of quantization levels during the course of training. Experiments on training deep neural networks show that our method can converge in much fewer communicated bits as compared to fixed quantization level setups, with little or no impact on training and test accuracy.
Joint Work with: Divyansh Jhunjhunwala, Advait Gadhikar, and Yonina Eldar.
- 15:00: Invited talk by Martin Jaggi (EPFL)
- Title: Communication-Efficient Distributed Deep Learning
Abstract
We discuss gradient compression methods for communication-efficient distributed training, in centrally-coordinated as well as fully decentralized scenarios.
In particular, we demonstrate that low-rank linear compressors applied on model differences can lead to fast encoding and decoding, as well as efficient aggregation between workers, while maintaining training and test accuracy. The key building blocks used are the linearity of the power iteration, applied to the fast-evolving matrix of gradients, paired with error-feedback.
We present empirical results on reduced training times for many neural network architectures with our open-source code, as well as theoretical convergence rates of the methods, applicable also for heterogeneous data, and asymptotically independent of the network topology and compression ratio.
Based on joint work with Sai Praneeth Karimireddy & Thijs Vogels.
- 15:30-16:00: Break
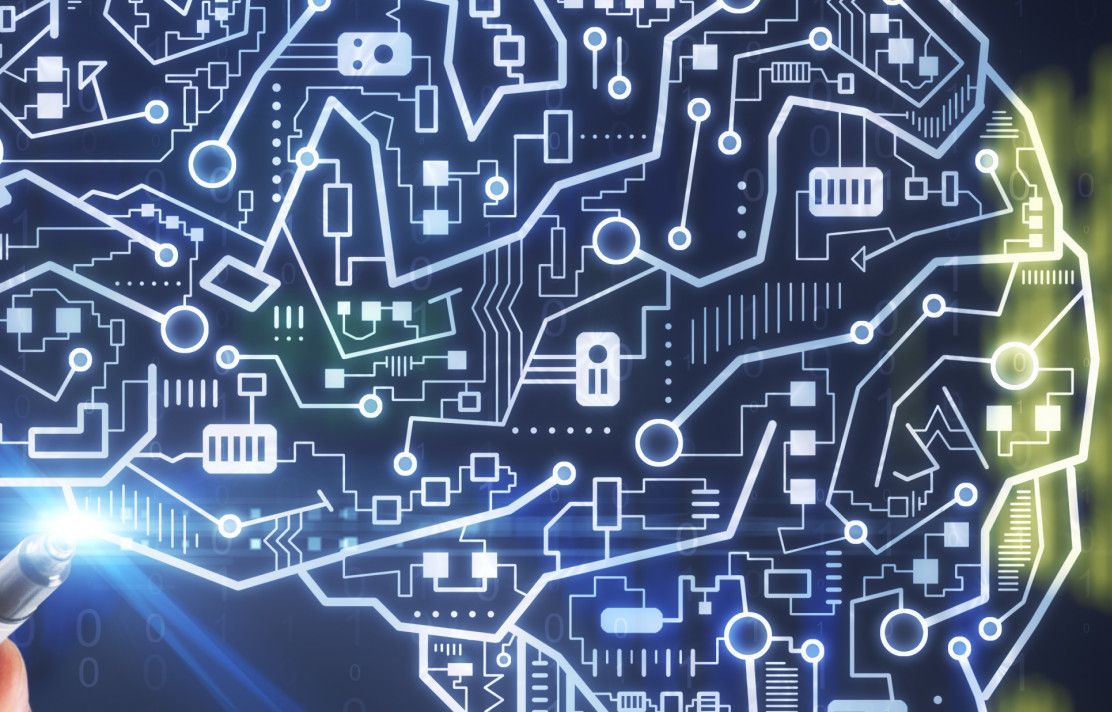
Past Events
LSIT 2021 Chairs
- Miguel Rodrigues (UCL)
- Osvaldo Simeone (KCL)
- Deniz Gunduz (Imperial)
Web Chair: Anouar Yatribi (Imperial)
Registration: Nitish Mital (Imperial)
Contact us:
a.yatribi@imperial.ac.uk
n.mital@imperial.ac.uk