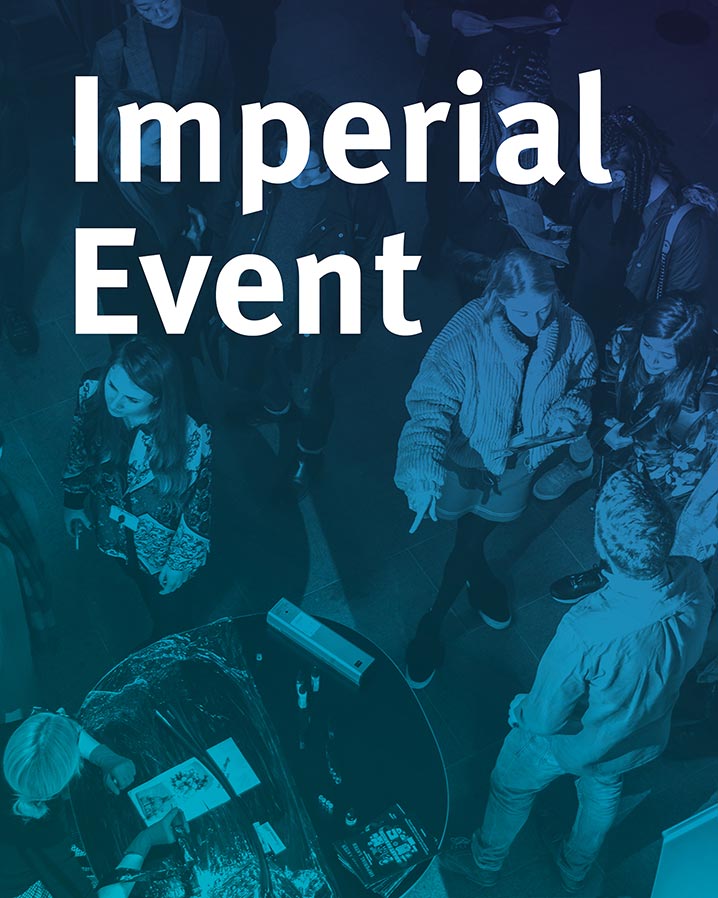
Title: Cell cycle gene regulation dynamics revealed by RNA velocity and deep-learning
Abstract: Despite the fact that the cell cycle is a highly studied fundamental process of life, a detailed quantitative understanding of gene regulation dynamics throughout the duration of the cell cycle is far from complete. Single-cell RNA-sequencing (scRNA-seq) technology gives access to these dynamics without externally perturbing the cell. By generating scRNA-seq libraries with high depth in mouse embryonic stem cells and human fibroblasts, we observe cycling patterns in the unspliced-spliced RNA space for every gene (RNA velocity). Since existing methods in scRNA-seq are not efficient to measure cycling gene dynamics, we propose a deep learning approach to fit these cycling patterns sorting single cells across the cell cycle. Combining RNA velocity with deep learning, we designed DeepCycle, a tool that assigns a continuous high-resolution cell cycle trajectory to single cells. DeepCycle allows the estimation of the gene activation-deactivation dynamics and results in fits of gene expression kinetics across the cell cycle. The link between cell cycle regulation and cell identity is not fully understood. Nonetheless, the cell cycle phases are deeply affected by the degree of stemness, for example, pluripotent and neural stem cells have short G1 phases, while committed cells extend their G1 phases and present overall longer cell cycles. Thanks to DeepCycle, we recapitulate these findings and uncover the underlying regulatory mechanisms, showing the different genes and transcription factors active in different cellular models across the cell cycle.