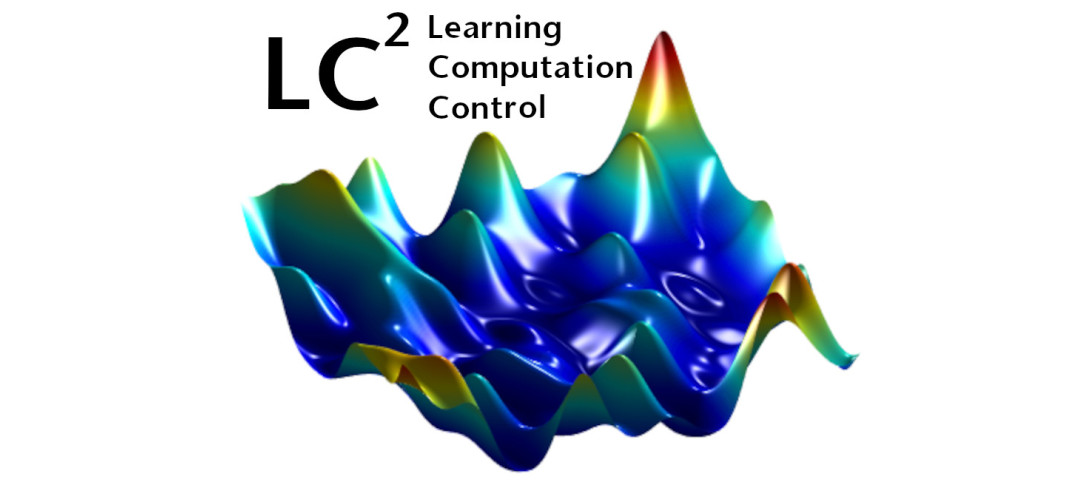
Abstract:
In this talk, we introduce and study a novel model-selection strategy for Bayesian learning, based on optimal transport, along with its associated predictive posterior law: the Wasserstein population barycenter of the posterior law over models. We first show how this estimator, termed Bayesian Wasserstein barycenter (BWB), arises naturally in a general, parameter-free Bayesian model-selection framework, when the considered Bayesian risk is the Wasserstein distance. We will then revise examples illustrating how the BWB extends some classic parametric and non-parametric selection strategies. We will conclude with a discussion about possible extensions and applications of the proposed BWB.
Joint work with J. Backhoff-Veraguas, J. Fontbona and G. Rios.
Based on:
J. Backhoff-Veraguas, J. Fontbona, G. Rios, F. Tobar (2022), Bayesian learning with Wasserstein barycenters, ESAIM: Probability and Statistics, vol. 26, p. 436-472.