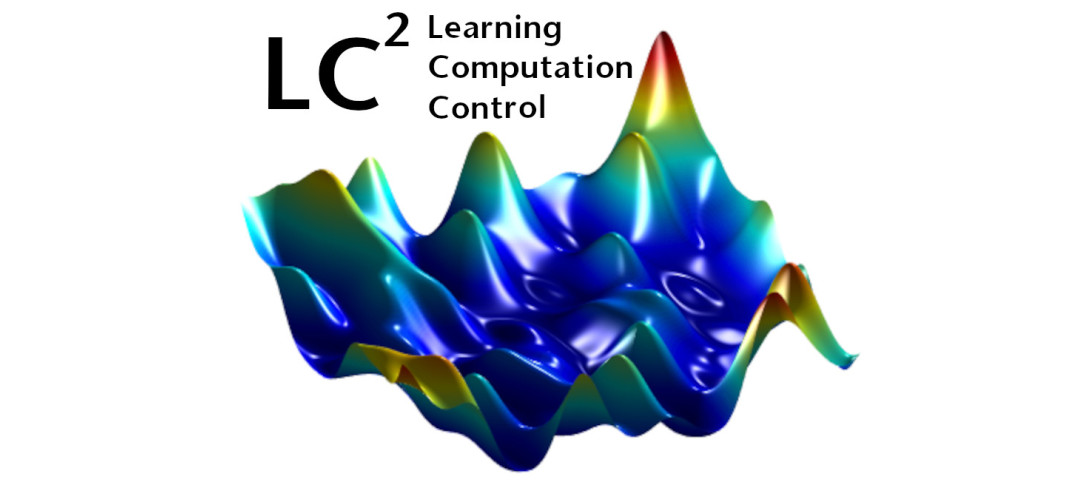
Abstract: System of interacting particles that display a wide variety of collective behaviors are ubiquitous in science and engineering, such as self-propelled particles, flocking of birds, and milling of fish. Modeling interacting particle systems by a system of differential equations plays an essential role in exploring how individual behavior engenders collective behaviors, which is one of the most fundamental and important problems in various disciplines. Although the recent theoretical and numerical study brings a flood of models that can reproduce many macroscopical collective patterns of the observed dynamics, the quantitative study towards matching the well-developed models to observational data is relatively scarce.
In this talk, we consider the data-driven discovery of systems of interacting particles with latent interaction kernels. We propose learning approaches that exploit the structure of the governing equation and cast the learning problem as an inverse problem. We discuss when this problem is well-posed, and construct estimators for the interaction kernels with provably good statistical and computational properties. We measure their performance on various examples, which include extensions to agent systems with different types of agents, second-order systems, and stochastic systems. We also conduct numerical experiments to test the large-time behavior of these systems, especially in the cases where they exhibit emergent behavior. This is based on the joint work with Fei Lu, Mauro Maggioni, Jinchao Feng and Charles Kulick.