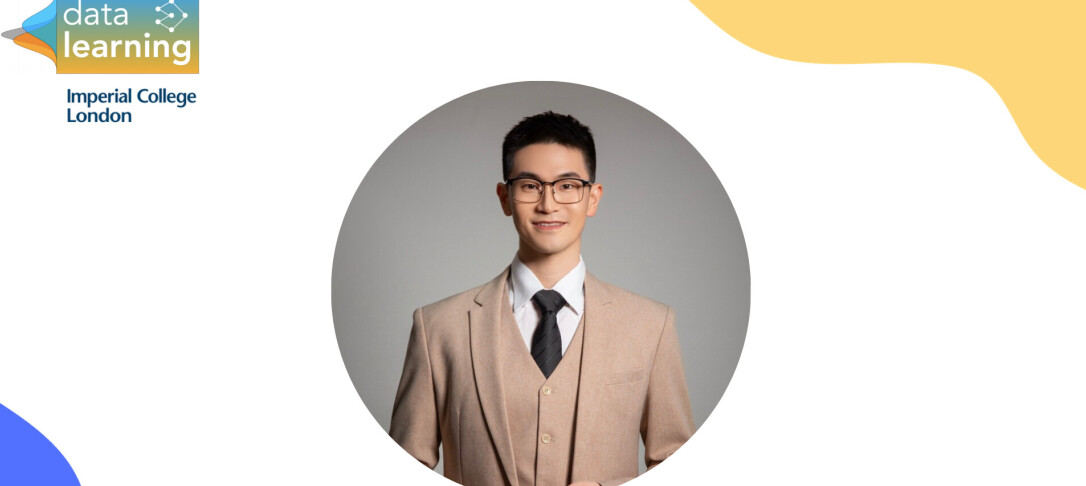
This Tuesday 14th November at 4 pm, the dataLearning seminars are hosting Wenbo Gong from Microsoft Cambridge. His talk is titled: “Causal Machine Learning with uncertainty in Directed Acyclic Graph (DAG) space”.
Join online: https://zoom.us/j/95291664305?pwd=b0lXeXNxbnllcjdCK2ZBaUs2WENyQT09
Abstract
The rapidly evolving field of causal machine learning is transforming the way we make data-driven decisions across a wide range of domains, including business engagement, medical treatment, and policy-making. A critical aspect of this process is the ability to infer the posterior distribution of causal models from observational data, which can help quantify the epistemic uncertainty and benefit downstream tasks. However, joint inference over Directed Acyclic Graphs (DAGs) space and nonlinear function parameters presents severe computational challenges. In the first part of this talk, we will discuss our approach, known as Deep End-to-End Causal Inference (DECI), which seamlessly integrates variational inference over DAG space through a regularizer with a nonlinear additive noise model. This innovative method allows for scalable and efficient approximate posterior inference over DAG space but suffers from the strong assumptions from the variational inference. In the second part of the talk, we will introduce our latest breakthrough: a scalable posterior inference over DAGs using Stochastic Gradient Markov Chain Monte Carlo (SG-MCMC). This overcomes the limitations of variational inference by directly drawing posterior samples from the DAG space and parameter space.
About the speaker
Wenbo Gong is a senior researcher at Microsoft Research Cambridge. He is interested in combining causality with deep generative models for solving real-world causal problems. Currently, he focuses on developing causal models for time series data and improving the posterior inference over DAGs. Before joining Microsoft, he finished his PhD at the University of Cambridge under the supervision of Jose Miguel Hernández Lobato.