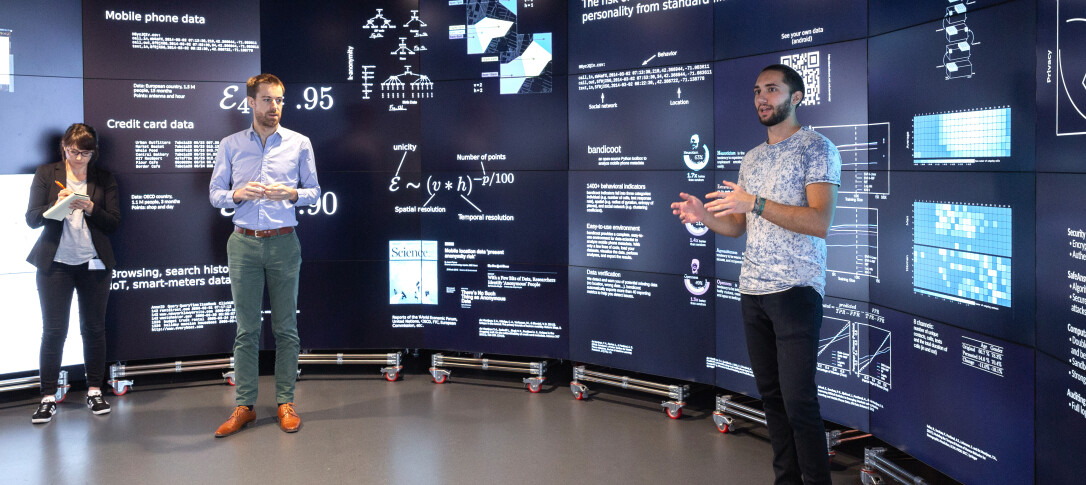
Abstract:
Differential privacy (DP) is widely regarded as gold standard privacy definition for machine learning and data analysis. The strong privacy protection can severely limit the accuracy of models trained under DP. Recent work has shown that the degradation of accuracy can be avoided by using fine-tuning of large pre-trained models. We explore the phenomenon further to understand its limits in terms of amount of data needed and the similarity of pre-training and target data, using different fine-tuning strategies, both in centralised and federated settings.
Bios:
Marlon Tobaben is a PhD student at the Department of Computer Science, University of Helsinki, supervised by Prof Antti Honkela and affiliated with the Finnish Centre of Artificial Intelligence (FCAI), a flagship of research excellence appointed by the Research Council of Finland. Marlon’s research focuses on differentially private deep and federated learning.
Antti Honkela is a Professor a Data Science (Machine Learning and AI) at the Department of Computer Science, University of Helsinki. He is the coordinating professor of Research Programme in Privacy-preserving and Secure AI at the Finnish Center for Artificial Intelligence (FCAI), a flagship of research excellence appointed by the Academy of Finland, and leader of the Privacy and infrastructures work package in European Lighthouse in Secure and Safe AI (ELSA), a European network of excellence in secure and safe AI. He serves in multiple advisory positions for the Finnish government in privacy of health data.