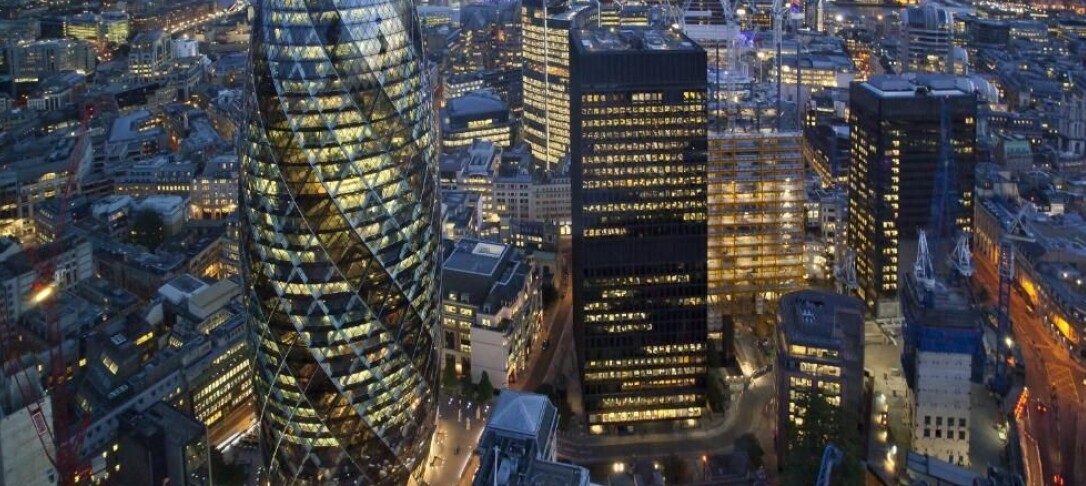
This workshop, held during the week of June 17, 2024, will bring together leading research groups in Math Finance from three of the world’s financial centers. The workshop will feature talks by academic researchers from Imperial College London, ETH Zurich, Chinese University of Hong Kong and the Hong Kong Polytechnic University.
Participants
Imperial:
Johannes Muhle-Karbe, Antoine (Jack) Jacquier, Eyal Neuman, Harry Zheng, Yonatan Shadmi, Pietro Siorpaes, Lukas Gonon, Yufei Zhang, David Itkin, Ofelia Bonesini, Ioannis Gasteratos, Damiano Brigo, Mikko Pakkanen, Philipp Jettkant, Anthony Coache, Nicola Muca Cirone, Konrad Müller, Will Turner, Francesco Piatti, Nikita Zozoulenko, Yun Zhao, Sturmius Tuschmann, Robert Boyce, Guangyi He, Ruben Wiedemann, Joseph Mulligan.
HK PolyU:
Min Dai, Kexin Chen, Zhaoli Jiang, Jiacheng Fan, Shuaijie Qian (HKUST).
CUHK:
Nan Chen, Chen Yang, Xuedong He, Lingfei Li, Yanwei Jia, Dohyun Ahn, Shuoqing Deng (HKUST).
ETH:
Beatrice Acciaio, Walter Farkas, Dylan Possamaï, Martin Schweizer, Josef Teichmann, Robert Crowell, Jakob Heiss, Songyan Hou, Evgeny Kolosov, Benjamin Kotlov, Florian Krach, Daniel Krsek, Patrick Lucescu, Tengyingzi Ma, Marco Rodrigues, Mateo Rodriguez, Chiara Rossata, Daria Sakhanda, Qinxin Yan.
Schedule
Monday June 17 | Tuesday June 18 | Wednesday June 19 | Thursday June 20 | ||
8:30-9:00 | Registration | 9:00-9:25 | Xuedong He | Mikko Pakkanen | |
9:00-9:10 | Opening remarks by Johannes Muhle-Karbe | 9:25-9:50 | Johannes Muhle-Karbe | Jiacheng Fan | Lingfei Li |
9:10-9:35 | Min Dai | 9:50-10:10 | Break | ||
9:35-10:00 | Josef Teichmann | 10:10-10:35 | Eyal Neuman | Yonatan Shadmi | Shuaijie Qian |
10:00-10:25 | Nan Chen | 10:35-11:00 | Yufei Zhang | Zhaoli Jiang | Pietro Siorpaes |
10:25-10:45 | Break | 11:00-11:10 | Break | ||
10:45-11:10 | Shuoqing Deng | 11:10-11:35 | Ahn Dohyun | Ofelia Bonesini | PhD talks Session 5 |
11:10-11:35 | Dylan Possamai | 11:35-12:00 | Ioannis Gasteratos | PhD talks Session 3 | |
11:35-12:00 | David Itkin | 12:00-12:25 | |||
12:00-13:15 | Lunch | 12:25-13:40 | Lunch | ||
13:15-13:40 | Kexin Chen | 13:40-14:05 | PhD Talks Session 2 | Lukas Gonon | |
13:40-14:05 | Harry Zheng | 14:05-14:30 | Chen Yang | ||
14:05-14:20 | Break | 14:30-14:45 | Social Program | Break | |
14:20-14:45 | Yanwei Jia | 14:45-15:25 | PhD talks Session 4 | ||
15:10-15:25 | Break | 15:25-15:35 | |||
15:25-15:50 | Antoine (Jack) Jacquier | 15:35-16:15 | |||
15:50-16:30 | PhD talks Session 1 |
PhD Talks
First Name | Family Name | Institute | |
Songyan | Hou | Imperial | Session 1 |
Robert | Crowell | ETH | |
Guangyi | He | Imperial | |
Jakob | Heiss | ETH | |
_________________________________________________________________________________________ | |||
Robert | Boyce | Imperial | Session 2 |
Sturmius | Tuschmann | Imperial | |
Florian | Krach | ETH | |
Nikita | Zozoulenko | Imperial | |
_________________________________________________________________________________________ | |||
Patrick | Lucescu | ETH | Session 3 |
Tengyingzi | Ma | ETH | |
Chiara | Rossato | ETH | |
Ruben | Wiedemann | Imperial | |
_________________________________________________________________________________________ | |||
Konrad | Müller | Imperial | Session 4 |
Francesco | Piatti | Imperial | |
Marco | Rodrigues | ETH | |
Mateo | Rodriguez | ETH | |
_________________________________________________________________________________________ | |||
Qinxin | Yan | ETH | Session 5 |
Yun | Zhao | Imperial | |
Daniel | Krsek | ETH | |
Joseph | Mulligan | Imperial |
Talk Information
Dohyun Ahn
Title: Efficient Simulation of Polyhedral Expectations with Applications to Finance
Abstract: We consider the problem of estimating the expectation over a convex polyhedron specified by a set of linear inequalities. This problem encompasses a multitude of financial applications including systemic risk quantification, exotic option pricing, and portfolio management. We particularly focus on the case where the target event is rare, which corresponds to extreme systemic failures, deep out-of-the-money options, and high target returns in the aforementioned applications, respectively. This rare-event setting renders the naive Monte Carlo method inefficient and requires the use of variance reduction techniques. To address this issue, we develop a novel and strongly efficient method for the computation of the said expectation in a general rare-event setting by exploiting the geometry of the target polyhedron and concentrating the sampling density almost within the polyhedron. The proposed method significantly outperforms the existing approaches in various numerical experiments in terms of accuracy and computational costs.
Robert Boyce
Title: Unwinding Stochastic Order Flow with Partial Information
Abstract: We consider the problem faced by a trader who wishes to unwind a stochastic order flow with uncertainty on the model parameterisation. Specifically, the order flow in this model is a stochastic process and it is influenced by unknown, intraday, low-frequency toxicity which reacts to the trader’s unwind strategy. As a result, the trader’s problem is an optimal liquidation problem, where the amount to liquidate is stochastic and evolves, and the adversarial effect of the order flow toxicity is unknown.
Kexin Chen
Title: Robust Dividend Policy: Equivalence of Epstein-Zin and Maenhout Preferences
Abstract: The classic optimal dividend problem aims to maximize the expected discounted dividend stream over the lifetime of a company. Since dividend payments are irreversible, this problem corresponds to a singular control problem with a risk-neutral utility function applied to the discounted dividend stream. In cases where the company’s surplus process encounters model ambiguity under the Brownian filtration, we explore robust dividend payment strategies in worst-case scenarios. We establish a connection between ambiguity aversion in a robust singular control problem and risk aversion in Epstein-Zin preferences. To do so, we first formulate the dividend problem as a recursive utility function with the EZ aggregator within a singular control framework. We investigate the existence and uniqueness of the EZ dividend problem. By employing Backward Stochastic Differential Equation (BSDE) representations where singular controls are involved in the generators of BSDEs, we demonstrate that the EZ formulation is equivalent to the maximin problem involving risk-neutral utility on the discounted dividend stream, incorporating Meanhout’s regularity that reflects investors’ ambiguity aversion. Considering the equivalent Meanhout’s preferences, we solve the robust dividend problem using a Hamilton-Jacobi-Bellman (HJB) approach combined with a variational inequality (VI). Our solution is obtained through a novel shooting method that simultaneously satisfies the VI and boundary conditions. This is a joint work with Kyunghyun Park and Hoi Ying Wong.
Nan Chen
Title: A Two Timescale Evolutionary Game Approach to Multi-Agent Reinforcement Learning and its Application in Algorithmic Collusion.
Abstract: In this paper, we propose a novel two timescale evolutionary game approach for solving general-sum multi-agent reinforcement learning (MARL) problems. Unlike existing literature that requires solving Nash equilibrium exactly or approximately in each learning episode, our new approach combines three key design components. First, we introduce a simple perturbed best response-based protocol for policy updates, avoiding the computationally expensive task of finding exact equilibria at each state. Second, agents use fictitious play to update their beliefs about other agents’ policies, relaxing the requirement for observable Q-values of all agents as in classical Nash Q-learning. Third, our algorithm updates policies, beliefs, and Q-values at two different time scales to address non-stationarity during learning. Importantly, our approach converges to approximate Nash equilibria for MARL problems without relying on global optimality or saddle point conditions, which are typically restrictive assumptions in the literature.
Additionally, AI-powered algorithms are increasingly used in marketplaces for pricing goods and services. However, regulators and academia express concerns about the potential collusion among these algorithms during strategic interactions. Most researchers rely on Q-learning to model pricing algorithm behavior, but this lacks convergence guarantees. Our approach provides a novel framework for algorithmic collusion studies.
This is a joint work with Ruixun Zhang and Yumin Xu (Peking University) and Mingyue Zhong (Tsinghua University).
Robert Crowell
Shuoqing Deng
Title: Stability of SMOT and the associated monotonicity principle.
Abstract: In this work, we shall study the stability of the supermartingale optimal transport(SMOT) problem. First, we consider the (lifted) canonical SMOT plans, and establish in particular the stability of the supermartingale shadow measures. Then, we shall focus on the more general SMOT problem, and establish the stability of the primal value and the transport plan. As a bi-product, we shall also study a supermartingale version of C-monotonicity principle for the Weak SMOT.
Based on joint works with Erhan Bayraktar(Michigan), Gaoyue Guo(CentraleSupelec) and Dominykas Norgilas(North Carolina State).
Ioannis Gasteratos
Title: Kolmogorov equations for Volterra processes
Abstract: We study a class of Stochastic Volterra Equations (SVEs) with multiplicative noise and convolution-type kernels. Our focus lies on rough volatility models and thus we allow for kernels that are singular at the origin. Working with carefully chosen Hilbert spaces, we rigorously establish a link between the solution of the SVE and the Markovian mild solution of an associated Stochastic Partial Differential Equation (SPDE). Our choice of a Hilbert space solution theory allows access to well-developed tools from stochastic calculus in infinite dimensions. In particular, we obtain an Itˆo formula for functionals of the solution to the SPDE and show that its law and (conditional) expectations solve infinite-dimensional Fokker-Planck and backward Kolmogorov equations respectively. Time permitting, we shall discuss potential applications of our results to optimal control and long-time behaviour of SVEs. This is joint work with Alexandre Pannier (Universit´e Paris Cit´e).
Lukas Gonon
Title: Quantum neural network expressivity
Abstract: Quantum neural networks have recently emerged as novel machine learning tools, suitable for implementations on quantum hardware. In this talk we present results on quantum neural network expressivity. We provide a link between required circuit complexity and desired approximation accuracy for functions with integrable Fourier transform. As an application example we consider option pricing in exponential Lévy models.
The talk is based on joint work with Antoine Jacquier.
Guangyi He
Xuedong He
Title: Reference-dependent asset pricing with a stochastic consumption-dividend ratio
Abstract: We study a discrete-time consumption-based capital asset pricing model under expectations-based reference-dependent preferences. More precisely, we consider an endowment economy populated by a representative agent who derives utility from current consumption and from gains and losses in consumption with respect to a forward-looking, stochastic reference point. First, we consider a general model in which the agent’s preferences include both contemporaneous gain-loss utility, that is, utility from the difference between current consumption and previously held expectations about current consumption, and prospective gain-loss utility, that is, utility from the difference between intertemporal beliefs about future consumption. A semi-closed form solution for equilibrium asset prices is derived for this case. We then specialize to a model in which the agent derives contemporaneous gain-loss utility only, obtaining equilibrium asset prices in closed from. Extensive numerical experiments show that, with reasonable values of risk aversion and loss aversion, our models can generate equity premia that match empirical estimates. Interestingly, the models turn out to be consistent with some well-known empirical facts, namely procyclical variation in the price-dividend ratio and countercyclical variation in the conditional expected equity premium and in the conditional volatility of the equity premium. Furthermore, we find that prospective gain-loss utility is necessary for the model to predict reasonable values of the price-dividend ratio.
This is a joint work with Luca De Gennaro Aquino, Moris S. Strub, and Yuting Yang
Songyan Hou
David Itkin
Antoine (Jack) Jacquier
Title: Wondering about the link between Optimal Transport and Quantum Adiabatic Theorem. Any help appreciated!
Yanwei Jia
Title: Continuous-time Risk-sensitive Reinforcement Learning via Quadratic Variation Penalty
Abstract: This paper studies continuous-time risk-sensitive reinforcement learning (RL) under the entropy-regularized, exploratory diffusion process formulation with the exponential form objective. The risk-sensitive objective arises either as the agent’s risk attitude or as a distributionally robust approach against the model uncertainty. Owing to the martingale perspective in Jia and Zhou (2023), the risk-sensitive RL problem is shown to be equivalent to ensuring the martingale property of a process involving both the value function and the q-function, augmented by an additional penalty term: the quadratic variation of the value process, capturing the variability of the value-to-go along the trajectory. This characterization allows for the straightforward adaptation of existing RL algorithms developed for non-risk-sensitive scenarios to incorporate risk sensitivity by adding the realized variance of the value process. Additionally, I highlight that the conventional policy gradient representation is inadequate for risk-sensitive problems due to the nonlinear nature of quadratic variation; however, q-learning offers a solution and extends to infinite horizon settings. Finally, I prove the convergence of the proposed algorithm for Merton’s investment problem and quantify the impact of temperature parameter on the behavior of the learning procedure. I also conduct simulation experiments to demonstrate how risk-sensitive RL improves the finite sample performance in the linear-quadratic control problem.
Zhaoli Jiang
Florian Krach
Abstract: In this talk we study the problem of (online) forecasting general stochastic processes using a path-dependent (PD) extension of the Neural Jump ODE (NJ-ODE) framework. While NJ-ODE was the first framework to establish convergence guarantees for the prediction of irregularly observed time series, these results were limited to data stemming from It\^o-diffusions with complete observations, in particular Markov processes, where all coordinates are observed simultaneously. In this work, we generalise these results to generic, possibly non-Markovian or discontinuous, stochastic processes with incomplete observations, by utilising the reconstruction properties of the signature transform. These theoretical results are supported by empirical studies. Applying the PD-NJ-ODE to the midprice forecasting problem in limit order books, once viewed as a regression and once as a classification problem, leads to state-of-the-art results. This is joint work with Marc Nübel and Josef Teichmann.
Daniel Krsek
Title: Randomisation with moral hazard: a path to existence of optimal contracts
Abstract: We discuss recent advancements in contracting theory. We consider a generic principal-agent problem in continuous time and introduce a framework in which the agent chooses relaxed controls. We characterize the agent’s value process as a solution to a BSDE driven by a martingale measure. This, in turn, allows us to employ compactification techniques and show the existence of optimal contracts, even with very general constraints imposed on the contract. The talk is based on joint work with Dylan Possamaï.
Lingfei Li
Title: Model-based reinforcement learning in diffusion environments
Abstract: We study continuous-time model-based reinforcement learning where the environment is modelled by a stochastic differential equation that defines a diffusion process. Instead of estimating the diffusion model by a statistical method such as maximum likelihood estimation (MLE), we pursue a value-aware approach for model learning that takes the structure of the decision problem into account, which has the potential of finding a better model for policy learning than the classical statistical approach when model misspecification exists. To perform value-aware estimation, we minimize the mismatch between the model-based value function and empirical rewards from the real environment and solve this problem based on numerically solving the value function partial differential equation. We develop a theory of our estimation approach. When the model is correctly specified, we establish convergence and asymptotic properties using the machinery of generalized method of moments. In the general case where model misspecification can exist, we obtain a representation that shows how the value function error is determined by the model error. We consider the problem of mean-variance portfolio selection to evaluate our method and demonstrate its advantages over model learning via MLE and the model-free approach in an empirical study.
Tengyingzi Ma
Johannes Muhle-Karbe
Title: Concave Cross Impact
Abstract: The price impact of large orders is well known to be a concave function of trade size.
We discuss how to extend models consistent with this “square-root law” to multivariate settings with
cross impact, where trading each asset also impacts the prices of the others. In this context, we derive
consistency conditions that rule out price manipulation, discuss how cross impact affects optimal
trading strategies, and illustrate these results using CFM metaorder data.
(Joint work in progress with Natascha Hey and Iacopo Mastromatteo)
Joseph Mulligan
Title: In-Sample and Out-of-Sample Sharpe Ratios for Linear Prediction Models.
Abstract: Before using a quantitative trading strategy, it should first be tested. This process has the potential to be fraught with issues which cause the in-sample performance to be significantly overestimated in relation to the out-of-sample performance of the strategy. We study the potential for overfitting when using a linear predictive model to trade and give analytical expressions for the in-sample and out-of-sample expected return and variance. We also show how these findings relate to the existing literature on estimation errors in portfolio optimisation and multiple testing.
Eyal Neumann
Title: Equilibrium in Functional Stochastic Games with Mean-Field Interaction
Abstract: We model the interaction between a slow institutional investor and a high-frequency trader as a stochastic multiperiod Stackelberg game.
The high-frequency trader exploits price information more frequently and is subject to periodic inventory constraints. We first derive the optimal strategy of the high-frequency trader given any admissible strategy of the institutional investor. Then, we solve the problem of the institutional investor given the optimal strategy of the high-frequency trader, in terms of the resolvent of a Fredholm integral equation, thus establishing the unique multi-period Stackelberg equilibrium of the game. Our results provide an explicit solution which shows that the high-frequency trader can adopt either predatory or cooperative strategies in each period, depending on the tradeoff between the order-flow and the trading signal. We also show that the institutional investor’s strategy is more profitable when the order-flow of the high-frequency trader is taken into account.
This is a joint work with Eduardo Abi Jaber and Moritz Voss.
Mikko Pakkanen
Title: A GMM approach to estimate the roughness of stochastic volatility
Abstract: I will present an approach to estimate log normal stochastic volatility models, including rough volatility models, using the generalised method of moments (GMM). In this GMM approach, estimation is done directly using realised measures (realised variance and the like), avoiding the biases that arise from treating the realised measures as proxies of spot volatility. I will also present asymptotic theory for the GMM estimator, permitting statistical inference, and apply the methodology to Oxford-Man Realised volatility data. Joint work with Anine Bolko, Kim Christensen and Bezirgen Veliyev.
Dylan Possamaï
Marco Rodrigues
Title: Reflections on BSDEs.
Abstract: We consider backward stochastic differential equations (BSDEs) and reflected BSDEs in a generality that allows for a unified study of certain discrete-time and continuous-time control problems with random time horizons. We provide well-posedness results for BSDEs and reflected BSDEs with optional obstacle processes, given appropriately weighted square-integrable data, and touch upon the corresponding second-order BSDEs. This is based on joint work with Dylan Possamaï.
Yonatan Shadmi
Pietro Siorpaes
Josef Teichmann
Title: Path dependence in Finance and Computer Science
Abstract: We analyze from a mathematical perspective the advantage of modelling dynamic phenomena on given state spaces by path dependent rather than state dependent characteristics. This is suggested by recent modelling successes in Finance or language generation.
Sturmius Tuschmann
Chen Yang
Title: Optimal Tax-Timing with Transaction Costs
Abstract: We develop a dynamic portfolio model incorporating capital gains tax (CGT), financial transaction tax, and transaction costs, where the tax amount is calculated at the end of each year. We find that transaction costs affect loss deferrals much more than gain deferrals, and a lower interest rate makes higher-wealth investors realize losses sooner but makes lower-wealth ones realize losses later. Our model can help explain the puzzle that even when investors face equal long-term/short-term CGT rates or almost zero interest rates, they may still defer realizing large capital losses. In addition, it provides several unique, empirically testable predictions and can shed light on recently proposed tax policy changes. This talk is based on a joint work with Min Dai, Yaoting Lei, and Hong Liu.
Yufei Zhang
Abstract: Static potential games, pioneered by Monderer and Shapley (1996), are non-cooperative games in which there exists an auxiliary function called static potential function, so that any player’s change in utility function upon unilaterally deviating from her policy can be evaluated through the change in the value of this potential function. The introduction of the potential function is powerful as it simplifies the otherwise challenging task of finding Nash equilibria for non-cooperative games: maximizers of potential functions lead to the game’s Nash equilibria.
In this talk, we propose an analogous and new framework called $\alpha$-potential game for dynamic $N$-player games, with the potential function in the static setting replaced by an $\alpha$-potential function. We present an analytical characterization of $\alpha$-potential functions for any dynamic game. For stochastic differential games in which the state dynamic is a controlled diffusion, $\alpha$ is explicitly identified in terms of the number of players, the choice of admissible strategies, and the intensity of interactions and the level of heterogeneity among players.
We provide detailed analysis for games with mean-field interactions, distributed games, and crowd aversion games, for which $\alpha$ is shown to decay to zero as the number of players goes to infinity, even with heterogeneity in state dynamics, cost functions, and admissible strategy classes. We also show $\alpha$ is capable of capturing the subtle difference between the open-loop and closed-loop strategies.
The talk is based on joint work with Xin Guo and Xinyu Li: https://arxiv.org/abs/2403.16962