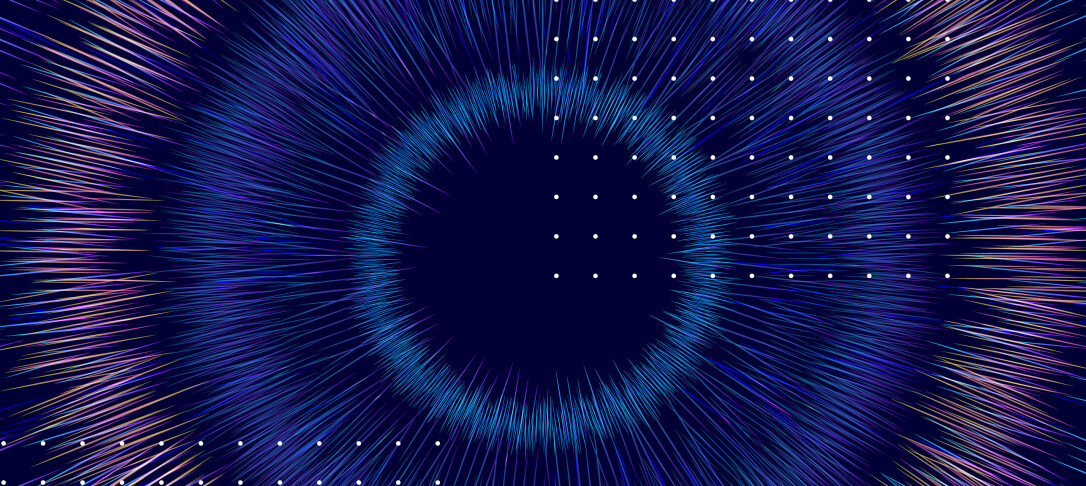
Abstract
In this talk Alessandra Di Pierro will discuss her work on kernel methods. Kernels in machine learning rely on a mathematical theory similar to the theory of quantum computation, as they both use Hilbert spaces for accomplishing their tasks.
At the base of this similarity is the notion of feature map, embedding input data from their original domain into a Hilbert space of higher dimensionality in order to find a linear separation among data. Quantum kernels are essentially obtained from the inner product functions in the Hilbert space of quantum states encoding classical data.
The use of this seemingly easy method actually brings about several problems in the choice of the appropriate embedding leading to an effective quantum advantage.
The talk will discuss some of these problems and present possible solutions and their applications.
Lunch
Please join us for lunch after the talk, 1pm to 2pm. Lunch will be served in the City and Guilds Level 2 Concourse area.
Join online
The online seminar will be hosted on Zoom. Passcode: $WsL2q