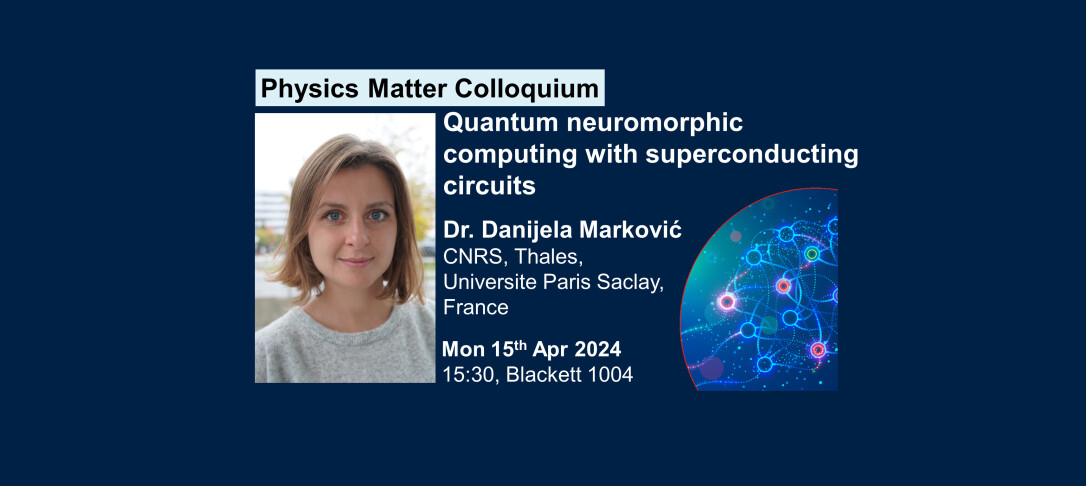
Join us for a colloquium with speaker Dr. Danijela Marković from the Laboratoire Albert Fert (CNRS,Thales), Université Paris/Saclay, France.
Quantum Neuromorphic Computing with Superconducting Circuits
Dr. Marković and her team are conducting fantastic research exploring the possibilities of using quantum phenomena such as superposition and entanglement to build a new class of machine learning hardware, using quantum superconducting circuits as a platform for quantum neural networks and reservoir computing.
Diverging from conventional quantum computing, Dr. Marković’s work examines ways we may circumvent some of the challenges facing the implementation of current quantum algorithms, taking inspiration from the noisy analog computing in biological brains to help overcome the challenges of error correction & fragile quantum states in conventional quantum computing.
Dr. Marković is an excellent speaker & her work is driving the direction of this emerging field. We will learn about physical systems which are promising hosts for quantum neuromorphic computing, how we can solve challenges in encoding computational AI problems in superconducting qubits and methods for constructing robust noise-tolerant output neurons to read out computational answers from quantum systems.
We look forward to seeing you there.
Abstract
Physical properties of quantum systems, such as superposition and entanglement, could allow them to exponentially accelerate some computations. Yet, quantum systems being noisy and their states fragile, execution of digital algorithms is out of reach of the existing devices. On the contrary, brain computes efficiently with weak analog signals, close to the noise level, through dynamical processes. Hence the idea behind quantum neuromorphic computing is to take inspiration from the brain, and use quantum dynamics and analog signals to compute with quantum systems [1,2]. We have performed simulations and experiments to demonstrate quantum neuromorphic computing with superconducting circuits [3]. Indeed, superconducting circuits allow to couple quantum oscillators in a parametric manner, using nonlinear elements based on Josephson junctions. Different parametric interactions can be driven and adjusted through drive parameters, that can be trained to obtain desired outputs. We first show that coupled quantum oscillators can transform the input data in a nonlinear manner which allows to classify it with a simple neural network architecture such as reservoir computing. Then we assess advantages of different variables that can be used as output neurons, i.e., occupation probabilities and emitted field quadratures. Finally, we compare different training methods, based on training Gaussian boson sampling distributions and variational methods.
[1] K. Fujii and K. Nakajima, Harnessing disordered-ensemble quantum dynamics for machine learning, Physical Review Applied 8, 024030 (2017).
[2] W. D. Kalfus, G. J. Ribeill, G. E. Rowlands, H. K. Krovi, T. A.Ohki, and L. C. G. Govia, Hilbert space as a computational resource in reservoir computing, Physical Review Research 4, 033007 (2022).
[3] Dudas, J., Carles, B., Plouet, E., Mizrahi, A., Grollier, J., & Marković, D., Quantum reservoir neural network implementation on coherently coupled quantum oscillators. Npj Quantum Information, 9, 64 (2023).