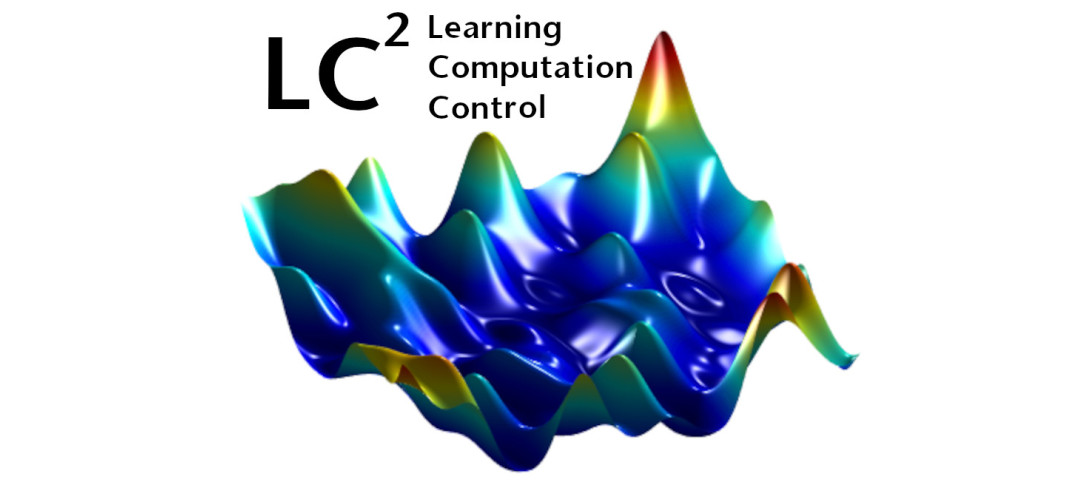
Abstract:
The concept of domain transformation is a common tool used in shape optimization via the method of mappings and in monolithic Arbitrary Lagrangian-Eulerian (ALE) formulations of Fluid-Structure Interaction (FSI) problems. Involving appropriate transformations, both can be formulated on a fixed reference domain. In order to obtain the transformations, one typically extends boundary or interface deformation to the interior of (parts of) the domain. If, at a discrete level, the transformed mesh degenerates, the arising systems of equations are not solvable, which yields numerical issues in performing shape optimization and simulating FSI. Several heuristic approaches based on partial differential equations (PDEs) have been proposed in the literature, including harmonic extension operators, linear elastic extensions (with space-dependent coefficients), biharmonic extension operators, and nonlinear techniques using the p-Laplace equations. In the scope of this talk, we discuss two choices of operators that are motivated by techniques from machine learning and require appropriately chosen neural network (NN) architectures. We consider a hybrid PDE-NN approach and a NN-corrected approach. Moreover, we discuss computational costs and generalizability of the proposed methods.