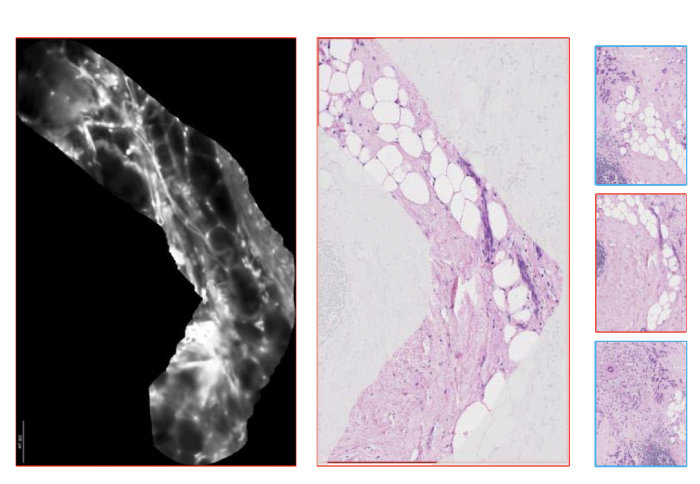

The Hamlyn Centre researchers proposed a transfer recurrent feature learning framework for classification tasks on pCLE videos to aid cancer diagnosis
Probe-based confocal laser endomicroscopy (pCLE) is an emerging tool for epithelial cancer diagnosis, which enables in-vivo microscopic imaging during endoscopic procedures and facilitates the development of automatic recognition algorithms to identify the status of tissues.
Using flexible fiber-bundle and miniaturized optics, it provides clinicians with real-time access to histological information during surgical procedures and has demonstrated promising sensitivities and specificities in various pre-clinical and clinical studies, including in the gastro-intestinal tract, breast and lungs.
Although pCLE enables the acquisition of in-vivo microscopic images that resemble closely to histology images, reliable diagnosis is still challenging for many clinicians who have little histopathology expertise and training.
Furthermore, the high variability in the appearances of pCLE images and the presence of atypical conditions makes it difficult to provide accurate diagnosis by manual identification.
A Transfer Recurrent Feature Learning (TRFL) Framework
The researchers at the Hamlyn Centre proposed a Transfer Recurrent Feature Learning (TRFL) framework for classification tasks on pCLE videos, aiming to aid the probe-based confocal laser endomicroscopy (pCLE) recognition.
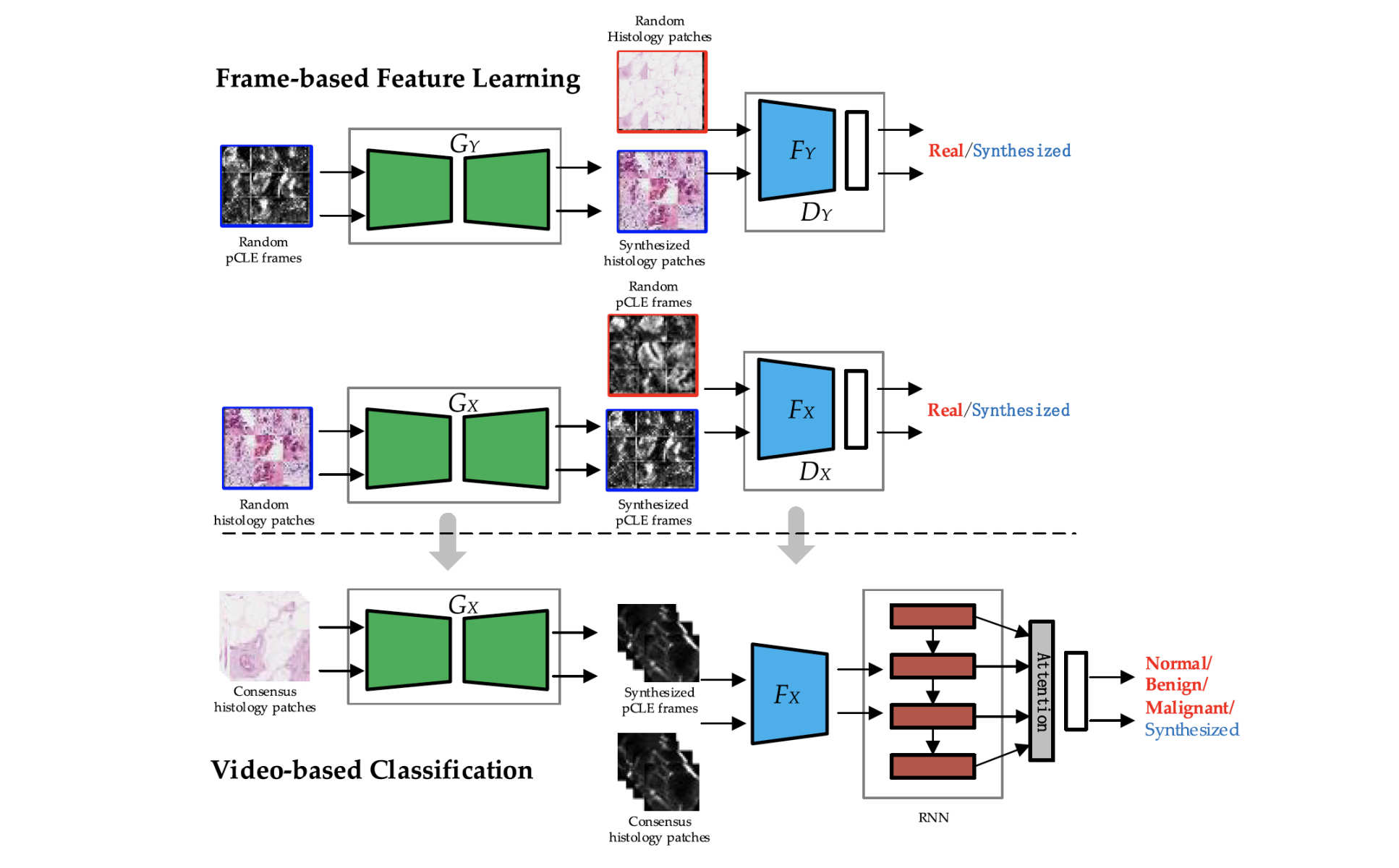
At the first stage, the discriminative feature of single pCLE frame was learned via generative adversarial networks based on both pCLE and histology modalities.
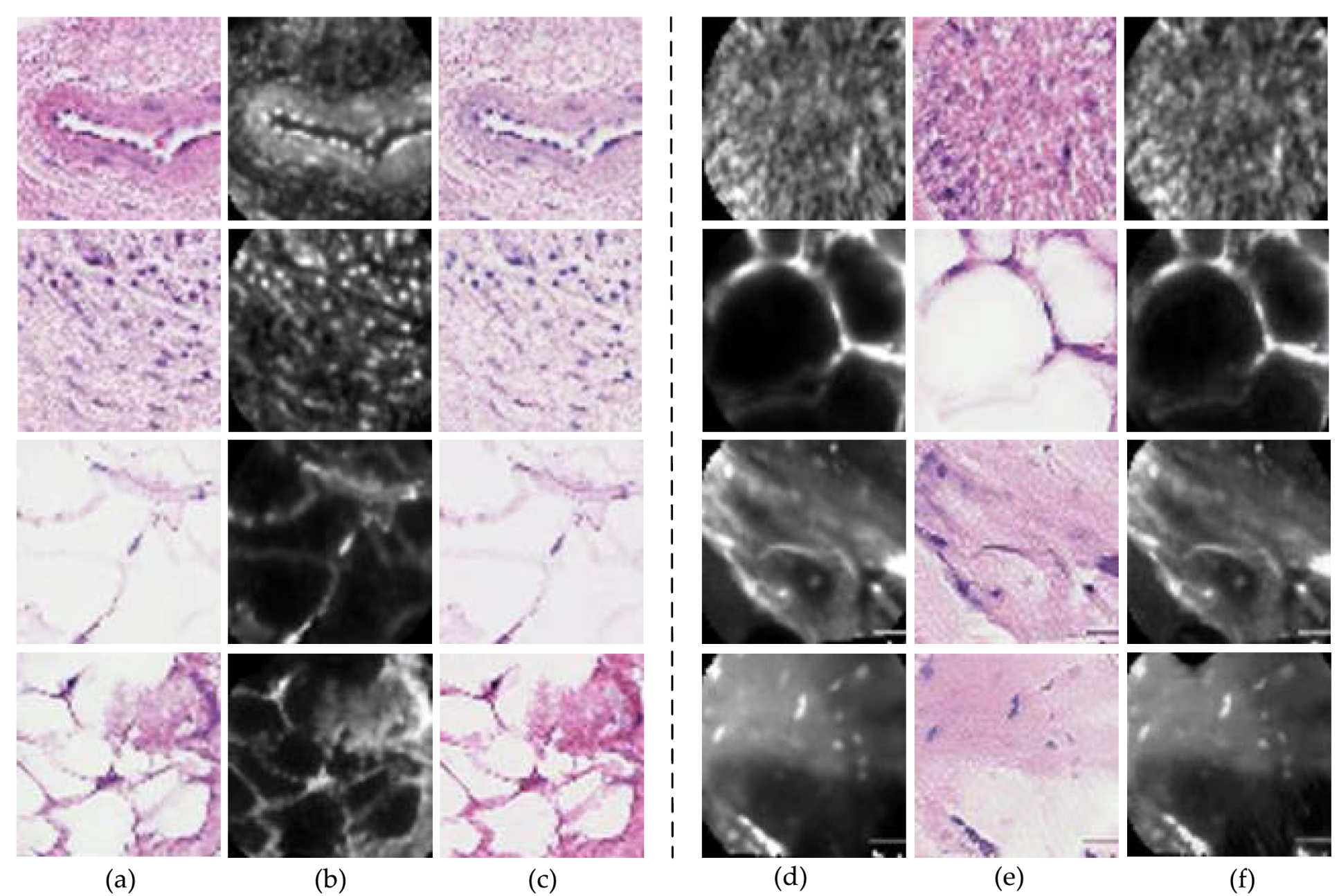
At the second stage, our researchers used recurrent neural networks to handle the vary length and irregular shape of field of view in pCLE mosaics, taking the frame-based features as input.
The experiments based on real pCLE data sets demonstrated that our approach outperforms, with statistical significance, state-of-the-art approaches. A binary classification accuracy of 84.1% has been achieved.
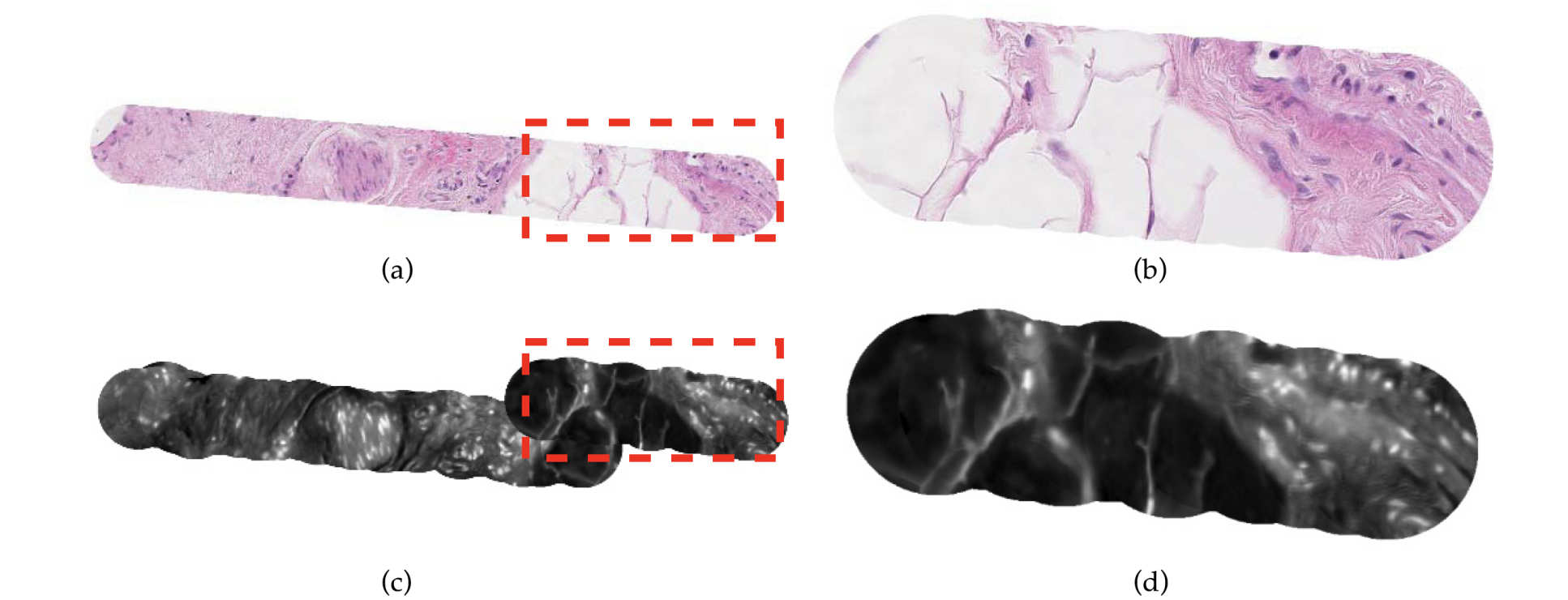
This research was supported by EPSRC Programme Grant “Micro-robotics for Surgery (EP/P012779/1)” and was published in IEEE Transactions on Medical Imaging ( and Guang-Zhong Yang, "Transfer Recurrent Feature Learning for Endomicroscopy Image Recognition").
Supporters
Article text (excluding photos or graphics) © Imperial College London.
Photos and graphics subject to third party copyright used with permission or © Imperial College London.
Reporter

Erh-Ya (Asa) Tsui
Enterprise

Contact details
Email: press.office@imperial.ac.uk
Show all stories by this author