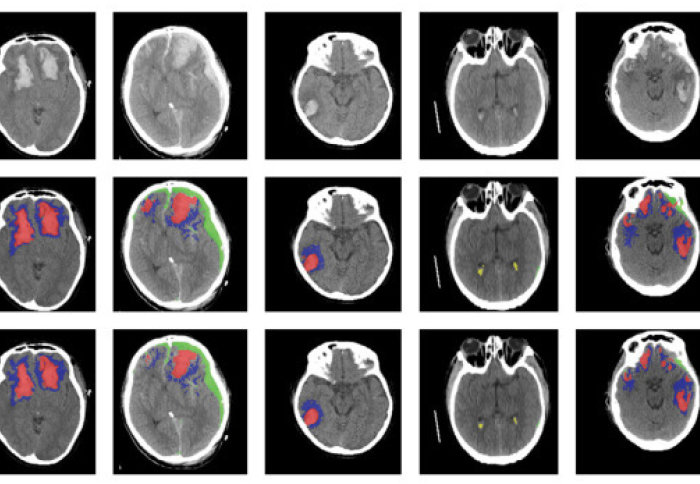

Researchers at Imperial College London and Cambridge have developed an AI algorithm that can detect and identify different types of brain injuries.
The researchers found that their machine learning algorithm was able to detect, segment, quantify and differentiate between types of brain lesions using images of CT scans.
Head injuries are a major health problem worldwide. Our artificial network and algorithm showed us that we can somewhat automate the diagnosis of different injury types to potentially help inform treatment in the future. Miguel Monteiro Department of Computing
The results, published in The Lancet Digital Health, could be used in large-scale research studies, for developing more personalised treatments for head injuries and, with further validation in clinical settings, could be useful in clinical scenarios where experts are in high demand.
Head injury is a global public health burden, affecting up to 60 million people each year. It is the leading cause of mortality in young adults. When a patient has had a head injury, they are usually sent for a CT scan to check for blood in or around the brain, and to help determine whether surgery is required.
PhD student Miguel Monteiro, who is co-first author on the study from Imperial’s Department of Computing, was a key developer of the AI tool. He said: “Head injuries are a major health problem worldwide. Our artificial network and algorithm showed us that we can somewhat automate the diagnosis of different injury types to potentially help inform treatment in the future.
“We’re currently only using the AI for research, but with proper validation, it could also be used in clinical scenarios like in resource-limited areas where there are few radiologists.”
Neural networks
AI in healthcare has so much potential, and we are only at the beginning of bringing this technology into clinical practice. Making sure that we address the right problems together with a careful clinical validation are key. Dr Ben Glocker Department of Computing
Different types of blood in or around the brain can lead to different patient outcomes, and radiologists will often make estimates in order to determine the best course of treatment, such as surgery or drugs.
Detailed assessment of a CT scan with annotations can take hours, especially in patients with more severe injuries, so the researchers designed an AI-based tool to automatically identify and quantify the different types of brain lesions from CT scans.
Co-senior author Professor David Menon from the University of Cambridge said: “CT is an incredibly important diagnostic tool, but it’s rarely used quantitatively. Often, much of the rich information available in a CT scan is missed, and as researchers, we know that the type, volume and location of a lesion on the brain are important to patient outcomes.”
Imperial researchers developed a machine learning tool based on an artificial neural network, developed by Imperial's Miguel Monteiro and Dr Ben Glocker. They trained the tool on more than 600 different CT scans showing brain lesions of different sizes and types, before validating the tool on an existing large dataset of CT scans.

The AI was able to classify individual parts of each image and tell whether it was normal or not. The researchers say this could be useful for future studies in how head injuries progress, since the AI may be more consistent than a human at detecting subtle changes over time.
The ability to automatically analyse large datasets could also enable the researchers to solve important clinical research questions that have previously been difficult to answer, including the determination of relevant features for prognosis, which in turn may help target therapies.
Miguel said: "It's exciting to be able to directly contribute to important clinical research questions with the algorithms that we develop, and it’s rewarding to see that our work has the potential to improve patient care.”
Co-senior author Dr Ben Glocker, also from Imperial’s Department of Computing, said: "This study is a prime example of how close collaboration between the engineering and clinical sciences can lead to real impact.
“AI in healthcare has so much potential, and we are only at the beginning of bringing this technology into clinical practice. Making sure that we address the right problems together with a careful clinical validation are key."
The researchers have made the Imperial software tool publicly available to the scientific community.
The research was funded by European Union 7th Framework Programme, Hannelore Kohl Stiftung, OneMind, NeuroTrauma Sciences, Integra Neurosciences, European Research Council Horizon 2020.
"Multiclass semantic segmentation and quantification of traumatic brain injury lesions on head CT using deep learning: an algorithm development and multicentre validation study" by Miguel Monteiro et al., published 14 May 2020 in The Lancet Digital Health.
This news story was adapted from a press release by the University of Cambridge.
Article text (excluding photos or graphics) available under an Attribution-NonCommercial-ShareAlike Creative Commons license.
Photos and graphics subject to third party copyright used with permission or © Imperial College London.
Reporter
Caroline Brogan
Communications Division

Contact details
Tel: +44 (0)20 7594 3415
Email: caroline.brogan@imperial.ac.uk
Show all stories by this author
Leave a comment
Your comment may be published, displaying your name as you provide it, unless you request otherwise. Your contact details will never be published.