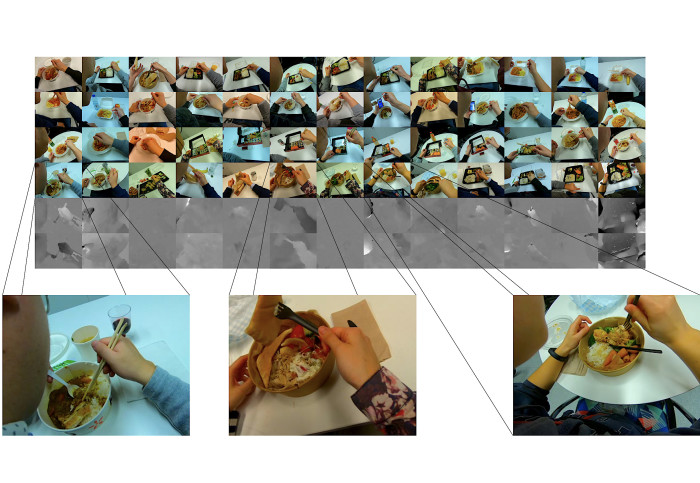

Our Hamlyn researchers proposed a deep-learning scheme for counting bites & recognising consumed food from videos, aiming to assist dietary monitoring
Current assessing dietary intake methods in epidemiological studies, such as 24-hour dietary recall and food frequency questionnaires (FFQs), are often inaccurate and inefficient.
It is because that these methods are predominantly based on self-reports, which depend on respondents’ memories and require intensive efforts to collect, process, and interpret.
Technological approaches are therefore emerging to provide objective dietary assessments. However, current technological approaches, such as sensing with eating action unit (EAU), are facing noticeable limitations when it comes to recognising food items and determining the exact food consumption.
A deep-learning scheme for counting bites and recognising consumed food from videos
Our research team at the Hamlyn Centre proposed a deep-learning scheme by combining both 2D convolutional neural networks (CNNs) and 3D CNNs in video recognition for tackling two unexplored targets in dietary intake assessment: 1) counting the number of bites within a time period from recorded dietary intake videos; 2) advancing food recognition to a much fine-grained level (consumed food recognition).
Using only egocentric dietary intake videos, this work aims to provide accurate estimation on individual dietary intake through recognising consumed food items and counting the number of bites taken.
It is worth to mention that this scheme is different from previous studies that rely on inertial sensing to count bites and only recognise visible food items but not consumed ones.
As an individual may not consume all food items visible in a meal, recognising those consumed food items is considered as valuable information for passive dietary monitoring.
Our researchers carried out the experimental assessment by monitoring the dietary intake process of 12 participants.
A total of 52 meals was captured throughout the monitoring process.
A new dataset that has 1,022 dietary intake video clips was constructed to validate the concept of bite counting and consumed food item recognition from egocentric videos.
A total of 66 unique food items, including food ingredients and drinks, were labelled in the dataset along with a total of 2,039 labelled bites.
Experimental results show that an end-to-end manner of bite counting and consumed food recognition is feasible with the use of deep neural networks.
Building on this work, our research team plan to expand current dataset as well as design upgraded models for dietary intake assessment.
This research was supported by the “Innovative Passive Dietary Monitoring Project (Opportunity ID: OPP1171395)” funded by the Bill & Melinda Gates Foundation (Jianing Qiu, Frank P.-W. Lo, Shuo Jiang, Ya-Yen Tsai, Yingnan Sun, and Benny Lo, "Counting Bites and Recognising Consumed Food from Videos for Passive Dietary Monitoring", IEEE Journal of Biomedical and Health Informatics , September 2020, PMID: 32897866).
Supporters
Article text (excluding photos or graphics) © Imperial College London.
Photos and graphics subject to third party copyright used with permission or © Imperial College London.
Reporter

Erh-Ya (Asa) Tsui
Enterprise

Contact details
Email: press.office@imperial.ac.uk
Show all stories by this author