

A new way of modelling air pollution can deliver more accurate and timely predictions.
Air pollution is a public health challenge threatening people and the environment – making timely and precise air quality measurements and predictions more crucial than ever.
To address this challenge, a team of researchers at the Department of Earth Science and Engineering and the Data Science Institute at Imperial College London have developed an innovative tool.
The new model, known as Dynamic Neural Assimilation (DyNA), combines the strengths of AI with data assimilation to provide fast and accurate forecasts of air pollution levels.
Findings, published in the journal Discover Applied Sciences, highlight a link between air pollutant data and nearby industrial activities, and demonstrate that the model is successful and more efficient than existing models at analysing air quality.
The new tool: DyNA
Older, more traditional tools capable of assessing air quality can provide accurate predictions, but they are often expensive and require lot of power and time to make predictions. DyNA, however, combines AI tools and data science to merge physics knowledge and data from sensors in a fast and reliable system.
DyNA, uses a customised Recurrent Neural Network (RNN) – a type of system that can keep track of data and use it to understand what could happen next, instead of processing one piece of information at a time, as more traditional systems do.
Coupled with data assimilation methods – which incorporate real-world observations in real-time – the DyNA tool provides a more reliable, efficient, and affordable way to accurately forecast air pollution levels and ingest data from air quality sensors.
In this way, DyNA can delve into past and present air quality data, learning patterns and using it to predict air quality in the future.
“Unlike more traditional, static models used to assess air quality, DyNA is dynamic and adaptable. It can fine-tune itself as soon as new data becomes available,” said Dr Rossella Arcucci, Senior Lecturer in Data Science and Machine Learning at the Department of Earth Science and Engineering, and Director of Research at the Data Science Institute.
“DyNA can use real-time information about air quality to improve the accuracy of its predictions. So, as soon as something that could affect air quality happens, DyNA quickly takes it into account and updates its predictions, helping ensure that we have the most up to date and reliable information about the air we breathe,” Dr Rossella Arcucci added.
Paving the way for a cleaner, healthier future
To validate the effectiveness of DyNA, the team of researchers trained and tested the model using real-world data obtained from air quality monitoring stations from the European Environment Agency in Italy.
For testing the system, they looked at air pollution data from 2003 to 2010 and identified that areas with monitoring sensors with lots of industrial activity tend to have higher pollution levels.
Findings therefore indicate that industrial activities were a major source of outdoor air pollution – suggesting the need for proactive monitoring, as well as strategies for mitigating pollution’s adverse effects on public health and the environment.
By providing quicker and more accurate air quality predictions, researchers hope that DyNA could help policymakers and environmental agencies make informed decisions and take the necessary actions to protect air quality - paving the way for a cleaner, healthier future.
Article text (excluding photos or graphics) © Imperial College London.
Photos and graphics subject to third party copyright used with permission or © Imperial College London.
Reporter
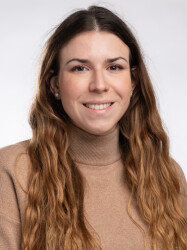
Diana Cano Bordajandi
Department of Earth Science & Engineering

Contact details
Email: press.office@imperial.ac.uk
Show all stories by this author