Download a PDF with the full list of our publications: Robot-Intelligence-Lab-Publications-2021.pdf
A comprehensive list can also be found at Google Scholar, or by searching for the publications of author Kormushev, Petar.
Results
- Showing results for:
- Reset all filters
Search results
-
Conference paperAhmadzadeh SR, Jamisola RS, Kormushev P, et al., 2014,
Learning Reactive Robot Behavior for Autonomous Valve Turning
-
Conference paperDallali H, Kormushev P, Tsagarakis N, et al., 2014,
Can Active Impedance Protect Robots from Landing Impact?
-
Conference paperJamisola RS, Kormushev P, Bicchi A, et al., 2014,
Haptic Exploration of Unknown Surfaces with Discontinuities
-
Conference paperAhmadzadeh SR, Carrera A, Leonetti M, et al., 2014,
Online Discovery of AUV Control Policies to Overcome Thruster Failures
-
Conference paperJamali N, Kormushev P, Caldwell DG, 2014,
Robot-Object Contact Perception using Symbolic Temporal Pattern Learning
-
Conference paperCarrera A, Karras G, Bechlioulis C, et al., 2014,
Improving a Learning by Demonstration framework for Intervention AUVs by means of an UVMS controller
-
Conference paperJamali N, Kormushev P, Ahmadzadeh SR, et al., 2014,
Covariance Analysis as a Measure of Policy Robustness in Reinforcement Learning
-
Conference paperCarrera A, Palomeras N, Ribas D, et al., 2014,
An Intervention-AUV learns how to perform an underwater valve turning
-
Conference paperCarrera A, Palomeras N, Hurtos N, et al., 2014,
Learning by demonstration applied to underwater intervention
-
Conference paperAhmadzadeh SR, Kormushev P, Caldwell DG, 2013,
Autonomous robotic valve turning: A hierarchical learning approach
, 2013 IEEE International Conference on Robotics and Automation (ICRA), Publisher: IEEE, Pages: 4629-4634, ISSN: 1050-4729Autonomous valve turning is an extremely challenging task for an Autonomous Underwater Vehicle (AUV). To resolve this challenge, this paper proposes a set of different computational techniques integrated in a three-layer hierarchical scheme. Each layer realizes specific subtasks to improve the persistent autonomy of the system. In the first layer, the robot acquires the motor skills of approaching and grasping the valve by kinesthetic teaching. A Reactive Fuzzy Decision Maker (RFDM) is devised in the second layer which reacts to the relative movement between the valve and the AUV, and alters the robot's movement accordingly. Apprenticeship learning method, implemented in the third layer, performs tuning of the RFDM based on expert knowledge. Although the long-term goal is to perform the valve turning task on a real AUV, as a first step the proposed approach is tested in a laboratory environment. © 2013 IEEE.
-
Conference paperKormushev P, Caldwell DG, 2013,
Improving the Energy Efficiency of Autonomous Underwater Vehicles by Learning to Model Disturbances
-
Conference paperAhmadzadeh SR, Kormushev P, Caldwell DG, 2013,
Interactive Robot Learning of Visuospatial Skills
-
Conference paperKarras GC, Bechlioulis CP, Leonetti M, et al., 2013,
On-Line Identification of Autonomous Underwater Vehicles through Global Derivative-Free Optimization
-
Conference paperAhmadzadeh SR, Kormushev P, Caldwell DG, 2013,
Visuospatial Skill Learning for Object Reconfiguration Tasks
-
Conference paperAhmadzadeh SR, Leonetti M, Kormushev P, 2013,
Online Direct Policy Search for Thruster Failure Recovery in Autonomous Underwater Vehicles
-
Conference paperJamali N, Kormushev P, Caldwell DG, 2013,
Contact State Estimation using Machine Learning
-
Conference paperKormushev P, Caldwell DG, 2013,
Comparative Evaluation of Reinforcement Learning with Scalar Rewards and Linear Regression with Multidimensional Feedback
-
Conference paperKormushev P, Caldwell DG, 2013,
Towards Improved AUV Control Through Learning of Periodic Signals
-
Conference paperLeonetti M, Ahmadzadeh SR, Kormushev P, 2013,
On-line Learning to Recover from Thruster Failures on Autonomous Underwater Vehicles
-
Conference paperKormushev P, Caldwell DG, 2013,
Reinforcement Learning with Heterogeneous Policy Representations
This data is extracted from the Web of Science and reproduced under a licence from Thomson Reuters. You may not copy or re-distribute this data in whole or in part without the written consent of the Science business of Thomson Reuters.
Contact us
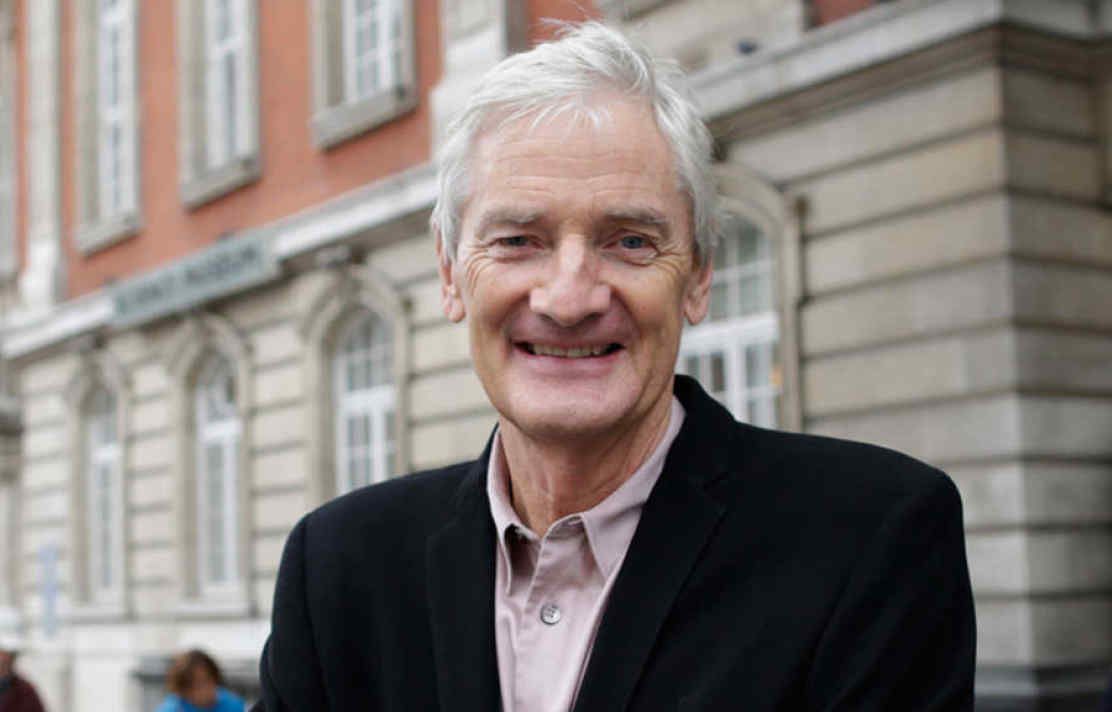