The redesign of the rubric can happen at three levels:
- Rethinking the criteria and to what extent they reflect what is expected of the students
In cases where AI use is allowed and encouraged you should look back on your assessment criteria to see whether any amendments need to be made. An important thing to consider is whether the use of AI means that students need to demonstrate new skills that have not been tested before, for example criticality (towards AI output) or reflection (on the output, own context etc). Similarly, if you choose to shift the focus on what is being assessed this needs to be reflected in the criteria. An example of a shift in focus is assessing the process not just product, also a good strategy to make your assessment less vulnerable in light of AI. Traditionally we assess the product, i.e. students work towards a piece of work that demonstrates their learning and judgments are based on that product. To make the assignment more AI proof you can focus on the process as well. An example of that is students showing their approaches to problem solving or showing their workings which can give insight into the thinking process that went into producing the final product. It is important to consider whether such a shift has a knock on effect on what is being assessed.
AI software can actually b quite helpful in helping you rethink your criteria. You can read more about how you can utilise AI for rethinking rubrics in this case study on the Anatomy of Assessment webpage.
- Rethinking the wording of the descriptors at each grade boundary
At times the same criteria can be assessed when the use of AI is encouraged but the degree to which these are demonstrated at each grade boundary might require further thinking. For example, an assessment that now asks to evaluate an AI output might require higher level of criticality so what constitutes pass, good and outstanding performance in that area might need changing.
- Redistributing the weighting within the marking scheme
Finally, you can rethink the weighting attached to different criteria. This could mean that lower level thinking skills that could easily be demonstrated by AI would attract minimal weighting. The higher order skills would be key to the task where the biggest weighting could be attached.
In each of these cases ensure that both staff and students understand the criteria well.
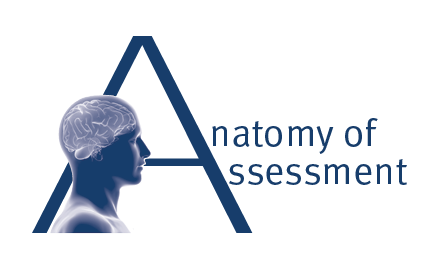