Performance analytics and data centric engineering
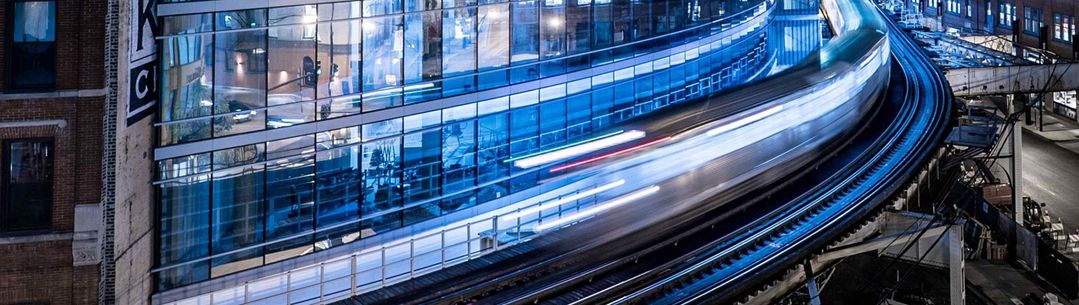
Major Themes
The users and suppliers of transport services generate an enormous amount of digital signs every time they buy a ticket, enter a station, close a train door or pass a trackside signal, for example. Planners, policy makers as well as researchers can learn a lot from these digital records, as the entire pattern of daily passenger movements and technological efficiency metrics are encapsulated in the resulting large-scale datasets. Our experience suggests, however, that transport agencies cannot fully utilise the new datasets that recently installed electronic fare payment or signally signalling systems, among many others, have made available. The primal reason why rich datasets are often stored for a while and then deleted without profitable information extraction is the absence of immediately available methods for specific types of quantitative analyses.
In the recent years, smart card and other automated data based research topics have become very popular in the academic sphere, thus resulting in a wide variety of research outcomes of mixed quality. Our research group contributes to this emerging field with (i) state-of-the-art econometric and causal inference methods that go beyond what black box data mining techniques can reveal, and (ii) the utilisation of over 20 years of experience we gained through benchmarking activities and collaborations with public transport operators all over the world. Our aim is to come up with research questions that are relevant for the industry and turn large-scale transport datasets into impactful results that the public transport sector can utilise in everyday decision making.
Recent Publications
Quantifying the effects of passenger-level heterogeneity on transit journey times
In this paper we apply flexible data-driven analysis methods on large scale mass transit data to identify areas for improvement in the engineering and operation of urban rail systems. Specifically, we use data from automated fare collection (AFC) and automated vehicle location (AVL) systems to obtain a more precise characterisation of the drivers of journey time variance on the London Underground, and thus an improved understanding of delay. Total journey times are decomposed via a probabilistic assignment algorithm and semiparametric regression is undertaken to disentangle the effects of passenger-specific travel characteristics from network related factors. For total journey times, we find that network characteristics, primarily train speeds and headways, represent the majority of journey time variance. However, within the typically twice as onerous access and egress time components, passenger-level heterogeneity is more influential. On average, we find that intra-passenger heterogeneity represents 6% and 19% of variance in access and egress times, respectively, and that inter-passenger effects have a similar or greater degree of influence than static network characteristics. The analysis shows that while network-specific characteristics are the primary drivers journey time variance in absolute terms, a non-trivial proportion of passenger-perceived variance would be influenced by passenger-specific characteristics. The findings have potential applications related to improving the understanding of passenger movements within stations, for example, the analysis can be used to assess the relative way-finding complexity of stations, which can in turn guide transit operators in the targeting of potential interventions.
Authors: Ramandeep Singh, Daniel J. Graham, Richard J. Anderson
Current status: Published in Data-Centric Engineering
Singh, R., Graham, D. J., & Anderson, R. J. (2020). Quantifying the effects of passenger-level heterogeneity on transit journey times. Data-Centric Engineering, 1.
Decomposing journey time variance on urban metro systems via semiparametric mixed methods
The availability of automated data for urban metro systems allows operators to accurately measure journey time reliability. However, there remains limited understanding of the causes of journey time variance and how journey time performance can be improved. In this paper, we present a semiparametric regression modelling framework to determine the underlying drivers of journey time variance in urban metro systems, using the London Underground as a case study. We merge train location and passenger trip data to decompose total journey times into three constituent parts: access times as passengers enter the system, on-train times, and egress times as passengers exit at their destinations. For each journey time component, we estimate non-linear functional relationships which we then use to derive elasticity estimates of journey times with respect to service supply and demand factors, including operational and physical characteristics of metros as well as passenger demand and passenger-specific travel characteristics.
We find that the static fixed physical characteristics of stations and routes have the greatest influence on journey time, followed by train speeds, and headways, for which the average elasticities of total journey time are −0.54 and 0.05, respectively. The results of our analysis could inform operators about where potential interventions should be targeted in order to improve journey time performance.
Authors: Ramandeep Singh, Daniel Hörcher, Daniel J. Graham, Richard J. Anderson
Current status: Published in Transporation Research Part C: Emerging Technologies
Singh, R., Hörcher, D., Graham, D. J., & Anderson, R. J. (2020). Decomposing journey times on urban metro systems via semiparametric mixed methods. Transportation Research Part C: Emerging Technologies, 114, 140-163.
A causal analysis of the impact of differential pricing on commuter trip scheduling in Hong Kong
This paper quantifies the causal impact of differential pricing on the trip-scheduling of regular commuters using the Mass Transit Railway (MTR) in Hong Kong. It does so by applying a difference-in-difference (DID) method to large scale smart card data before and after the introduction of the Early Bird Discount (EBD) pricing intervention. We find statistically significant but small effects of the EBD in the form of earlier departure times. Leveraging the granularity of the data, we also allow for the treatment effect to vary over observed travel characteristics. Our empirical results suggest that fares and crowding are the key determinants of commuter responsiveness to the EBD policy.
Authors: Anupriya, Daniel J. Graham, Daniel Hörcher, Richard J. Anderson, and Prateek Bansal
Current status: Published in Transportation Research Part A: Policy and Practice
Anupriya, Graham, D. J., Hörcher, D., Anderson, R. J., & Bansal, P. (2020). Quantifying the ex-post causal impact of differential pricing on commuter trip scheduling in Hong Kong. Transportation Research Part A: Policy and Practice, 141, 16-34.